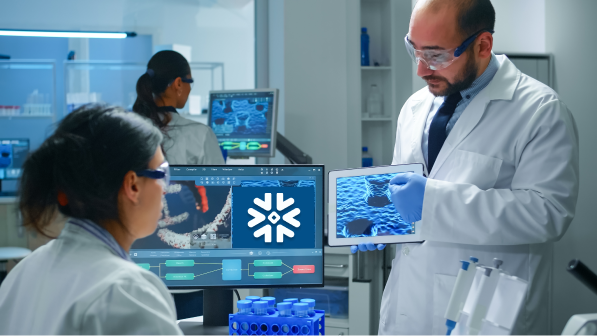
Snowflake is a cloud-based data warehousing and analytics platform at the forefront of the data revolution. It is changing the game for healthcare organizations by making it easier to leverage the power of AI and machine learning (AI/ML) to turn data into meaningful insights. Here are some of the ways that Snowflake and NutaNXT are powering next-generation AI healthcare analytics systems:
1. One Place to Instantly Access All Relevant Data
One of the most significant challenges in healthcare analytics is the time-consuming process of locating and requesting access to relevant data. This data can be stored in various systems, including electronic health records (EHRs), clinical decision support systems (CDSSs), and medical imaging systems. Snowflake provides a single access point to a vast global network of trusted data. This feature enables data scientists and analysts to work more efficiently.
To support Snowflake-powered AI healthcare analytics systems, NutaNXT offers the performance and scalability required. NutaNXT can scale to any size and can handle even the most demanding workloads.
Diverse Data, Unified Platform
Snowflake supports a wide variety of data types, including structured, semi-structured (JSON, Avro, ORC, Parquet, or XML), and unstructured data. You can bring almost all types of healthcare data into their AI models without the need for complex data pipelines. From electronic health records to unstructured text feedback from patients or other data sources, NutaNXT can seamlessly integrate with Snowflake’s flexibility.
Augmenting Model Performance with Shared Data
Snowflake has enabled NutaNXT to augment its AI models by leveraging shared data sets from its business ecosystem and third-party data available on the Snowflake Marketplace.
By collaborating on data sharing, NutaNXT is able to tap into a wealth of information to power its AI healthcare analytics systems. It’s not just about the data that NutaNXT possesses but also the data that others bring to the table, all effortlessly integrated within Snowflake.
2. Fast Processing Engine with Minimal Operational Complexity
In the realm of AI healthcare analytics, the need for speed and operational simplicity cannot be overstated. The capacity to swiftly transform raw data into valuable insights can significantly impact patient care, research, and decision-making. With the advanced features of Snowflake, we’ve elevated our data processing capabilities to new heights.
Transforming Data into ML-Powered Insights with Snowpark
Snowflake’s remarkable feature is its ability to turn data into ML-powered insights using a language of your choice, thanks to Snowpark. We’ve harnessed this capability to seamlessly perform complex data transformations, run machine learning algorithms, and generate actionable insights.
The flexibility in language choice empowers our data scientists to work with the programming language they find most comfortable, ensuring a streamlined analytics pipeline. Whether it’s Python, R, or any other language, Snowpark facilitates a unified approach to data processing.
Speeding Up Python Workflows with Anaconda Integration
For organizations like ours, heavily reliant on Python-based workflows for data analysis and modeling, Snowflake’s Anaconda integration is a game-changer. Snowflake comes pre-installed with a wide array of open-source libraries commonly used in Python data science and analytics. This eliminates the need for time-consuming installations and setup, allowing our data experts to dive right into their work.
Furthermore, Snowflake offers seamless dependency management, ensuring that the necessary libraries and packages are readily available. This simplifies the development process and ensures our Python-based workflows run at peak performance.
Scaling Processing to Any Amount of Data or Users
In healthcare analytics, the ability to scale processing to handle large datasets and accommodate numerous users is indispensable. Snowflake’s elastic and performant multi-cluster compute architecture provides us with the scalability we require.
We can dynamically adjust our processing resources to match the demands of our analytics workloads. Whether we’re dealing with a small dataset or a vast trove of healthcare records, Snowflake’s architecture adapts seamlessly, preventing any performance bottlenecks.
3. Single Platform to Unify Teams and Tools Around Data
In the pursuit of next-generation AI healthcare analytics systems, achieving synergy among teams and tools is crucial. One should appreciate the value of having a unified platform where data experts can collaborate seamlessly and where tools can work harmoniously to harness the full potential of data. With Snowflake, this can be achieved effectively.
Developing in Your Preferred Environment with Snowpark ML APIs
One of the remarkable features of Snowflake is its flexibility in development. Data scientists and analysts can develop models and algorithms in their preferred environments, whether it’s a notebook or an integrated development environment (IDE). This flexibility empowers them to use the tools and languages they are most comfortable with, making the development process smoother and more efficient.
What sets Snowflake apart is its ability to securely process these models at scale using Snowpark ML APIs. This ensures that no matter where the models are developed, they can be seamlessly integrated into Snowflake’s ecosystem, facilitating a cohesive and comprehensive approach to data analysis.
Scalable and Secure ML Inference with Snowflake
In the realm of AI healthcare analytics, it’s not just about model development; it’s also about deploying those models efficiently and securely. Snowflake excels in this regard. Snowflake’s capabilities can be leveraged to run scalable and secure ML inference.
Models can be run inside Snowflake as User-Defined Functions (UDFs), or they can communicate with a secure model endpoint using External Functions. This approach allows for the scaling of ML inference operations as needed while ensuring the security and integrity of models and data.
Effortlessly Making Model Results Accessible
The ultimate goal of AI healthcare analytics is to make ML-driven insights readily available to the teams and applications that need them. Snowflake facilitates this effortlessly. Models can be published directly in Snowflake, creating a central repository for insights.
Healthcare professionals, researchers, and decision-makers can easily access and act on the results of AI models, enhancing the quality of patient care and the efficiency of healthcare operations. The data doesn’t just stay within the domain of data scientists; it becomes a valuable resource for the entire healthcare ecosystem.
Conclusion
Snowflake provides NutaNXT with the agility and security they need to develop, deploy, and share AI models seamlessly. ML-driven healthcare analytics can be at the forefront of innovation if they are developed in a preferred environment, run scalable and secure inferences, and make model results intuitively accessible. With Snowflake, it’s not just about building a data-driven ecosystem; it’s about creating a new standard for healthcare analytics that is efficient, collaborative, and impactful.
As the journey to power the next generation of AI healthcare analytics systems through Snowflake continues, it’s evident that it’s not just about improving healthcare data analysis; it’s about redefining what’s possible in data-driven healthcare.