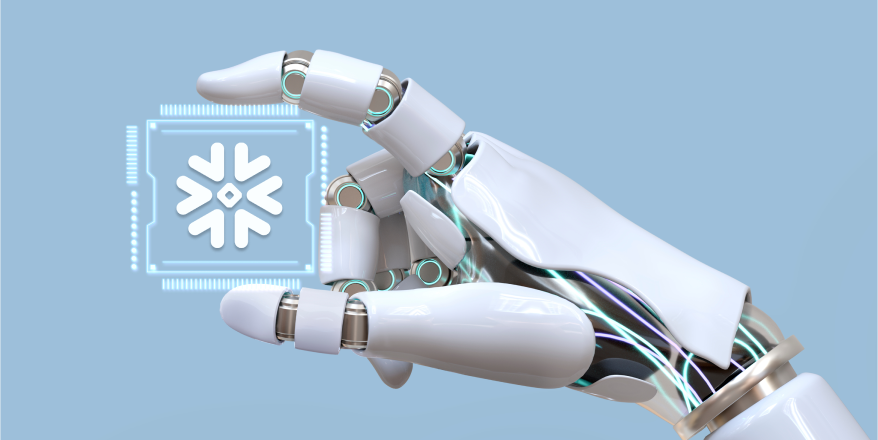
Integrating AI with data warehousing solutions like Snowflake empowers organizations to derive meaningful insights, enhance decision-making, and unlock new possibilities for innovation.
Significance of Data in AI Solutions
Data plays a vital role in training and deploying AI models. AI models perform better when they are trained on more data. It is, however, challenging for organizations that are new to AI to manage and utilize large datasets. Cloud data platforms like Snowflake offer several advantages for AI solutions. With its scalable infrastructure and flexible pricing models, Snowflake allows organizations to store and process gigantic amounts of data efficiently. Its built-in security features also address data privacy concerns.
An Overview of Snowflake
Snowflake is a renowned cloud-based data warehousing solution. Its strengths lie in its simplicity, massive workload handling capacity, and stringent data security and compliance measures.
Snowflake offers several features that are vital for AI solutions:
- Scalability: Snowflake’s elastic architecture makes it ideal for training and deploying AI models on large datasets.
- Performance: Snowflake’s unique architecture allows fast query execution and parallel processing, enabling quick insights and real-time analytics.
- Security: Snowflake employs robust security measures to protect sensitive data, including encryption, role-based access control, and auditing.
- Data sharing and data marketplace: Snowflake enables easy data sharing between organizations, thus fostering collaboration.
Use cases of organizations utilizing Snowflake in AI/ML projects
Numerous organizations across industries have successfully integrated Snowflake into their AI and machine learning (ML) projects. Healthcare companies have utilized Snowflake’s scalability and security features to analyze vast amounts of patient data and generate personalized treatment plans. Retail businesses have leveraged Snowflake to analyze customer behavior and optimize inventory management, leading to improved sales and customer satisfaction.
Financial institutions can use Snowflake to store and analyze large volumes of transaction data to detect fraudulent transactions, assess risk, and personalize financial products and services. Snowflake can also be used to store and analyze sensor data from manufacturing equipment to predict when maintenance is needed. AI and ML models can be trained on this data to automatically inspect products for defects or identify patterns and anomalies that may indicate fraud.
Integrating AI with Snowflake
Integrating AI models with Snowflake involves connecting the AI platform and Snowflake’s data warehouse. This connection allows AI models to access and utilize data stored in Snowflake for training and prediction. However, some of the possible challenges when integrating AI with Snowflake include:
- Data quality: AI models are only as good as the data on which they are trained. The data in Snowflake must be of high quality and accurate.
- Model performance: AI models can be computationally expensive to train and deploy. It is critical to choose the proper AI algorithm and optimize it.
- Security: AI models are susceptible to hacking. It is necessary to put security measures in place to safeguard AI models from unauthorized access.
Building an AI Solution with Snowflake: A Step-by-Step Guide
Building an AI solution with Snowflake requires a structured approach encompassing key stages.
- Clearly defining the problem statement and objectives helps organizations focus their AI efforts and align them with business goals.
- Designing the AI model involves selecting the appropriate algorithm and performing feature selection and data preparation to ensure the model’s accuracy and efficiency.
- Snowflake plays a crucial role in managing data for AI solutions. It involves ingesting data into Snowflake and performing data transformation and preparation using Snowflake’s capabilities.
- Snowflake’s efficient data processing capabilities enable organizations to train AI models using the data stored in Snowflake, ensuring accurate and reliable predictions.
- Deploying the AI model involves integrating it into the existing infrastructure and monitoring its performance to ensure it continues to deliver accurate results.
- Once deployed, the AI model can generate valuable insights that can guide decision-making and drive business actions.
- Snowflake’s capabilities facilitate continuous model improvement by enabling organizations to retrain their AI models with new data, ensuring the models remain effective over time.
Best Practices for Building AI Solutions with Snowflake
Adhering to best practices ensures the successful implementation of AI solutions with Snowflake.
- Ensuring data quality and integrity in Snowflake: Establishing proper data governance practices and quality checks within Snowflake promotes data integrity.
- Efficient data transformation and preparation: Optimizing data transformation and preparation processes within Snowflake can significantly improve the efficiency of AI model development and deployment.
- Implementing robust security practices: Snowflake’s built-in security features need to be properly configured and enhanced as per organizational security policies.
- Ensuring scalability and performance optimization: Properly scaling and optimizing Snowflake resources helps maintain performance and cost efficiencies as AI workloads grow.
- Continuous monitoring and improvement of AI models: Monitoring AI models after deployment and incorporating user feedback helps identify areas for improvement.
Future Trends: AI and Snowflake
The future holds exciting possibilities for the integration of AI and data warehousing solutions like Snowflake.
- AI and Data Warehousing Evolution: AI will transform business operations with advanced analytics and automation. Snowflake will evolve to meet growing AI demands.
- Emerging Tech in AI and Data Management: Expect advances in AI algorithms like deep learning and improved data management techniques.
- Snowflake’s Role in Future AI: Snowflake’s flexibility, scalability, and security make it adaptable and contribute to evolving AI technologies.
Conclusion
When combined, AI and Snowflake present a formidable toolset for organizations. By harnessing the scalability, performance, and security offered by Snowflake, businesses can unlock innovative, data-driven solutions. To fully benefit, however, organizations need a proactive approach to this integration, tapping into the vast potential for growth, cost savings, and insights.