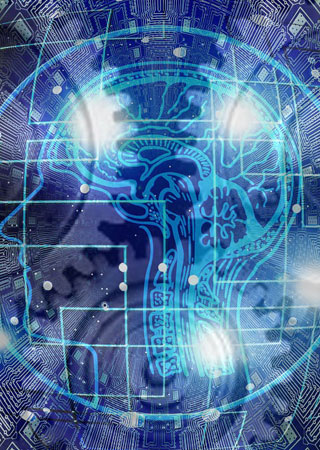
Automation of credit scoring and loan acquisition processes using AI/ML
Business Problem
One of our B2C consumer FinTech customers wanted to build an AI/ML based customer qualification and scoring model to make real-time decisions for underwriting and issuing new loans within seconds of cycle time.
Traditional credit scoring methods need data on credit history and loan repayment history for outstanding lines of credit. Consumers in middle to low-income segments are unlikely to have enough credit history and sometimes may have no credit history. However, these segments were key growth markets for new loan origination for which building a new credit scoring model was important. AI/ML based credit scoring models can factor in much more data to arrive at not only a better picture of creditworthiness, but also predict loan default risk based on the data.
Our Solution
We successfully built a new credit scoring model and customer scorecard from scratch. The foremost challenge with this project was the lack of any precedence or historical data or benchmark use case to model our algorithm. One of our key objectives was to help build an improved customer qualification and credit decision process, which can provide a significant advantage to any company in the unsecured lending business. We built a process which identified new data sources which could bring in millions of data points to be analysed by our custom statistical algorithms. Additionally, our models were able to accurately analyse behavioural attributes of to significantly improve the loan customer qualification process.
We helped our client to fetch data from various structured and unstructured databases using data engineering tools and build a proprietary credit scoring mechanism using AI/ML techniques to score customers. The model factored in more than 1800 internal and external data points and was developed using AI, ML, advanced data analytics and statistical modelling to benchmark customers on the basis of which lending decisions are taken by the risk functions and underwriting teams.
Business Impact
The customized scorecards helped to increase top line and bottom line by cross selling, up selling. The client saw a significant reduction in customer dropouts. By using our ML model, the client was able to make a real-time decision of underwriting a quick loan in less than 7-8 seconds.
Underwriters are able to reject loan applicants from borrowers who were qualified to be riskier than their credit scores implied and as a result they were able to improve the quality of the loan portfolio and avoid losses they would have otherwise incurred if loans were issued to these borrowers. Based on the success of our project, our client currently distributes more than 60,000 loans a month using our ML-based credit scorecard and disbursed more than 500 million USD in loans so far.
Our implementation helped the client to significantly improve the quality of the loan book and see much lower delinquency rates (up to 25% lower) for loans issued to first time borrowers.