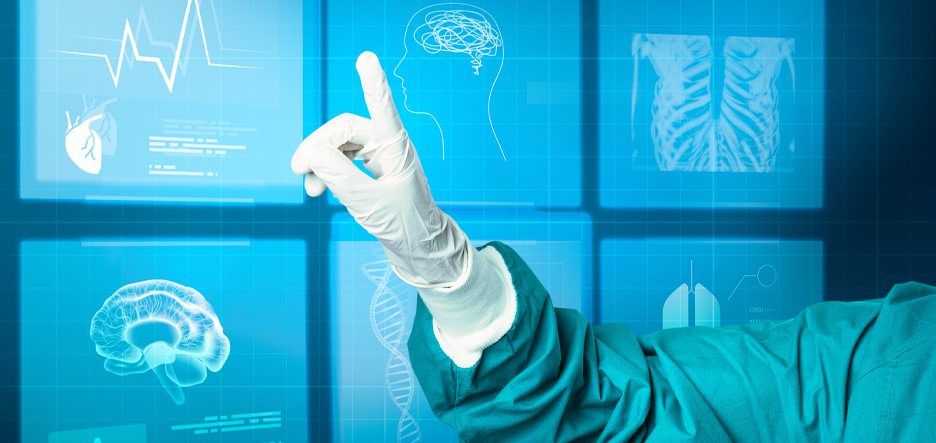
Technology and medicine have gone hand and hand for many years. From telemedicine to artificial intelligence, robotic surgery, and 3D printing, technology is revolutionizing the healthcare industry. Medical devices are poised to alter the healthcare experience for patients, caregivers, and insurance companies as they progress into smart, connected, and scalable solutions. Cloud, mobility, augmented reality, and artificial intelligence/machine learning are upending traditional business structures and solution methodologies.
According to GlobalData, the artificial intelligence (AI)/machine learning (ML) platform market is expected to grow to $52 billion in 2024, up from $29 billion in 2019. With the uncertainty brought by the Covid-19 pandemic, the medical technology industry has been adopting new technologies quickly. As a result, some of the largest investors in AI and ML belong to the MedTech industry, including GE Healthcare, Medtronic, and Philips. Bringing AI and machine learning into the historically conservative realm of medical technology, offers the potential to improve present ways of diagnosing, managing, and treating a wide range of diseases and health conditions. Furthermore, as big data becomes more accessible and controllable, it has now become possible to analyze, process, and discover new patterns in medical data, particularly when paired with machine learning algorithms.
Traditionally, medical device software was a stand-alone code with two distinct purposes: medical and non-medical. The first is defined as “Software as a Medical Device,” which is described as “software that is designed to help a medical purpose or functionality and performs this function without being part of any hardware device.” The second is described as ‘software in a medical device,’ consisting of device drivers, firmware, connection apps, and other system software packages, is a different medical device software.
In this article, we will discuss SaMD and the benefits of implementing AI into it.
The medical purpose for SaMDs are as follows:
- A physiological state, such as diseases or injuries, is investigated and diagnosed.
- (Disease) treatment and mitigation, or relief (of injury)
- Physiological state prognosis/prediction
- Body vitals, such as heart rate and blood oxygen, are monitored, and life support is provided.
SaMD is built based on below functional categories that are aligned with the purpose as described earlier
- By linking data streams from another diagnostic or therapeutic device, you can diagnose, treat, or reduce disease.
- To promote increased medical use, disease risk prediction, definitive diagnosis, and identification of illness early.
- Provide aggregated patient medical history information to inform treatment options.
Creating value through AI/ML technologies in SaMDs
For data aggregating, collection, and management for clinical efficiency and productivity, SaMD can provide remote monitoring for in-patient and out-patient use cases. Sensor data collection, networking, cloud integration, and user application development are all examples of these. In SaMDs, AI/ML technologies can be utilised to support diagnostic (both imaging and non-imaging) and prescriptive use cases, which are frequently based on aggregated multi-modal data.
Hence to develop a smart SaMD, the critical components required are:
- Preprocessing and tailoring data across modalities using a data management pipeline
- Feature extraction and algorithm training
- Interpretability, assessment, and inference
Medtronic is currently concentrating on incorporating AI into its existing surgery sectors, such as enhanced image processing, robotics, and navigation, as well as improving remote patient monitoring recruitment. In 2021, the company plans to release more than ten of its pipeline items in essential remote solutions and innovations. A pre-procedure platform called UNiD ASI, which can employ predictive modeling algorithms to model digital spine reconstruction and perform measurements is one of Medtronic’s primary items to implement in surgery. Medicrea GROUP, a medical equipment firm established in France that supplies surgical solutions to neurosurgeons and orthopedic surgeons, developed UNiD ASI.
Philips has chosen to concentrate on a distinct market trend, namely AI diagnosis with increased precision and process informatics. Phillips reached a $2.8 billion merger agreement with BioTelemetry in December 2020. BioTelemetry specializes in cardiac diagnostics, wearable heart monitors, AI-based data processing, and outside-the-hospital monitoring.
But not all companies are joining the bandwagon because embedding AI/ML in SaMD has challenges associated with it.
- Traditional medical device laws were not built to accommodate adaptive AI/ML technologies.
- As fresh data is acquired, adjustments and enhancements owing to AI/ML technologies may result in modifications.
- From locked to constantly adaptable algorithms, AI/ML based SaMD exists on this spectrum. The test methodologies, quality, and applicability of the dataset used for testing and the algorithm’s training technique all influence the rigor of performance evaluation for both locked and continuously adaptive algorithms.
The above stated issues can be solved by using a Total Product Life Cycle (TPLC) strategy, which allows for a quick cycle of product improvement and allows these devices to improve while still offering effective safeguards.
The AI and machine learning sectors are exploding, and the Covid-19 pandemic has only expedited this tendency. Large corporations have been aggressively investing in and deploying AI and machine learning technology, which will surely boost the MedTech business and set new norms.